Improved wind-speed forecasts can help urban power generation, according to new Concordia research
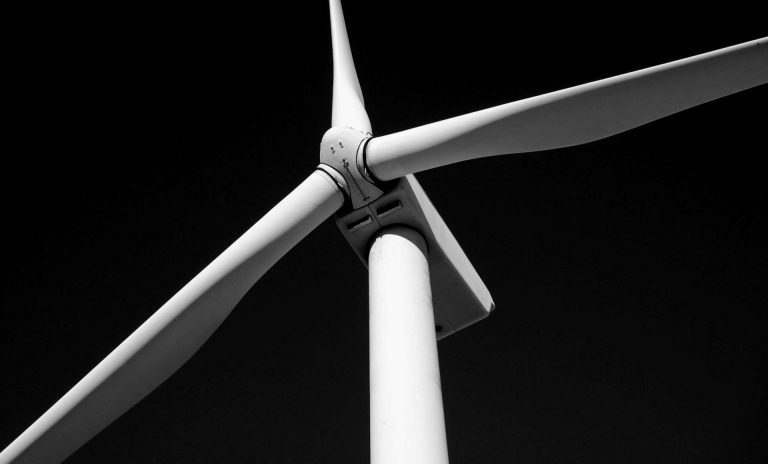
Wind-generated electricity will play a vital role in global energy capacity as humanity pivots away from fossil fuels towards renewables. Wind speed is a key parameter in estimating wind energy potentials in a location. But predicting wind speed is a difficult task. That presents a challenge for utilities that need to rely on reliable estimates to power their grids.
Several models already predict wind speed with varying degrees of accuracy and reliability. In a Concordia-led study published in the journal Energies, researchers from the Department of Building, Civil and Environmental Engineering at the Gina Cody School of Engineering and Computer Science present a hybrid method that integrates multiple types of models.
The researchers say this hybrid method can improve forecasting accuracy by up to 30%. The project began as part of a thesis by then-PhD student Navid Shirzadi (PhD 23). Shirzadi was looking at designing a microgrid for Concordia University’s downtown Montreal campus.
The new approach uses data analysis and outputs from two sources: a Weibull probability distribution and a numerical weather prediction (NWP). The Weibull distribution in this case predicts wind speed probabilities based on historical data and other variables. By contrast, the NWP uses physical principles to predict future behaviour based on a large, complex algorithm. NWPs are commonly used by weather services and apps for hourly and daily forecasts.
While these models have their strengths, Shirzadi says they have significant limitations when trying to apply them to power generation.
“The stochastic behaviour of renewables and their fluctuations make designing and operating a microgrid very challenging,” he explains.
“There is no special pattern or seasonality that you can capture easily. We need a very strong model in order to predict wind power generation.”

The researchers first fused the Weibull probabilities into a Long Short-Term Memory (LTSM) model. An LTSM is a type of recurrent neural network that is especially useful for time-series analysis. The researchers then added data from the NWP to further strengthen their model.
The initial hybridization between the LSTM and the Weibull distribution yielded promising results, Shirzadi says. These results were strengthened with the addition of the NWP: errors in forecasting were reduced by 32 per cent when compared to non-hybridized LTSM predictions over a 48-hour horizon.
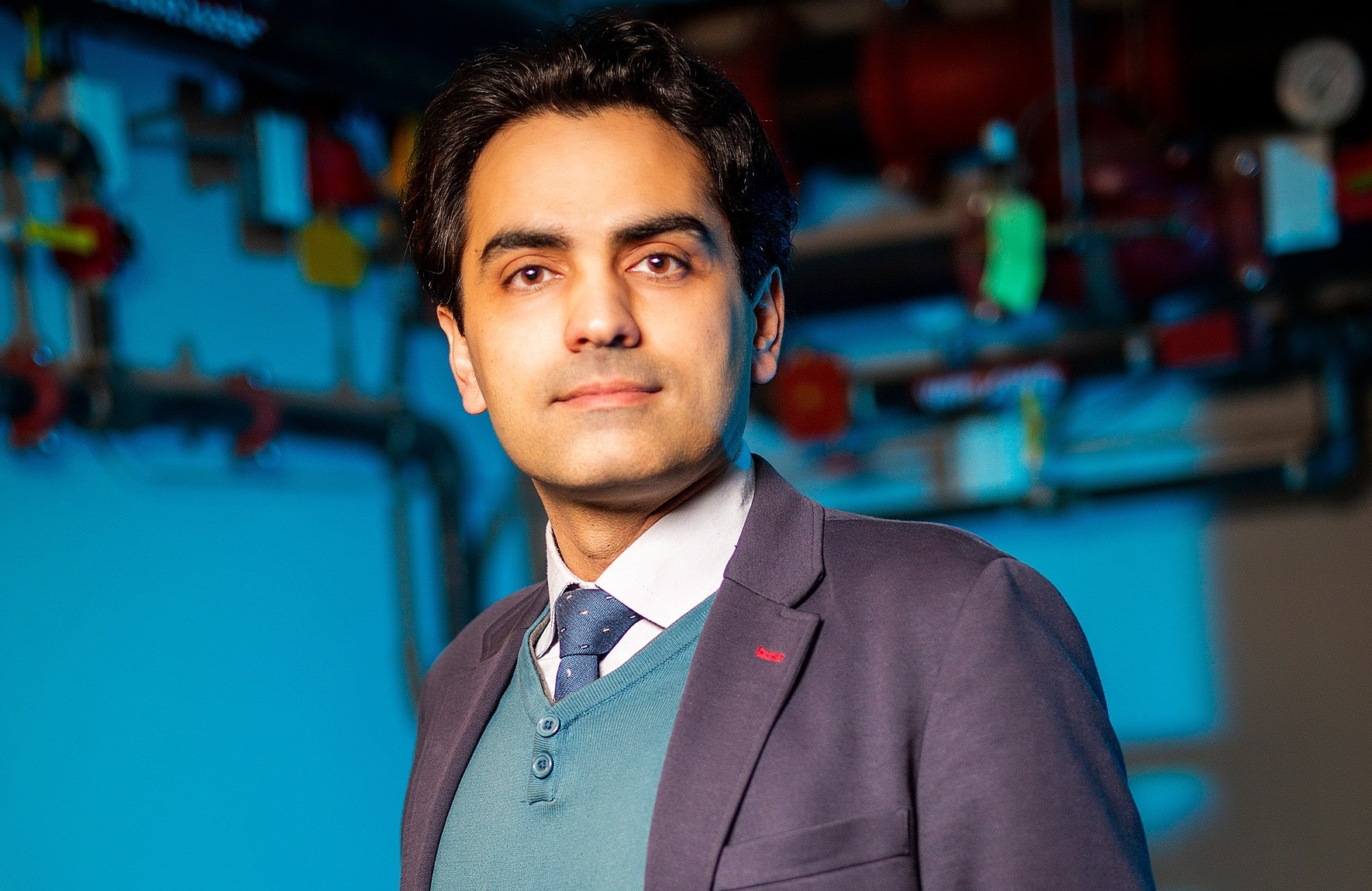
Gusts for growth
While wind power continues to grow worldwide, much more remains to be done before achieving the international net zero by 2050 scenario. According to the International Energy Agency, achieving net zero emissions by mid-century requires that we generate 7,400 TWh from wind alone by the end of this decade. Global wind electricity generation stands at 2100 TWh today, meaning solid year-on-year growth in investment will be needed for the foreseeable future.
Associate professor and co-author Fuzhan Nasiri says he believes this research—along with other research being produced by members of the university’s Next Generation Cities Institute and Volt-age initiative—could contribute to Concordia’s decarbonization efforts. Concordia is moving toward its own Net Zero Emissions by 2050 commitments, positioning the university as a Canadian leader.
“We must diversify our energy sources. Local capacities are a part of that,” Nasiri explains.
“We cannot rely solely on the existing grid, which can be vulnerable to natural disasters like ice storms. It adds a buffer during power outages. So, it is extremely important to have some precise idea about wind speed to see which area has the highest potential for a turbine’s operational efficiency.”
Ursula Eicker, Canada Excellence Research Chair in Smart, Sustainable and Resilient Cities and Communities, and Shirzadi’s main supervisor, emphasizes that “energy management increasingly gains in importance, as intermittent renewables need to match the demand all the time. Accurate wind speed prediction is the basis to best predict the variable power generation and adapt the loads and storage usage.”
Concordia’s CERC team postdoctoral researchers Ramanunni Menon and Pilar Monsalvete, as well as Anton Kaifel at the Centre for Solar Energy and Hydrogen Research in Stuttgart, Germany, also contributed to the study.
This research received funding from the NSERC Discovery grant program and the Tri-Agency Institutional Program Secretariat.
Read the cited paper: “Smart Urban Wind Power Forecasting: Integrating Weibull Distribution, Recurrent Neural Networks, and Numerical Weather Prediction”