Abobakr Al-Sakkaf is a an architect and urban planner specializing in sustainability and energy efficiency in heritage buildings. He has extensive experience in developing models to assess the sustainability and energy performance of these structures in Canada and internationally. Currently, he is creating a framework to advance technology solutions to assess the conditions and retrofit of heritage buildings. Abobakr holds a PhD in Building Engineering, a Master’s in Architectural Engineering, and a Bachelor’s double major in Architecture and Urban Planning. He has published over 100 papers on sustainability, architectural engineering, green building, construction management, MCDM, LCA, BIM, AI, Machine Learning and IoT applications in building engineering.
Postdoctoral fellows
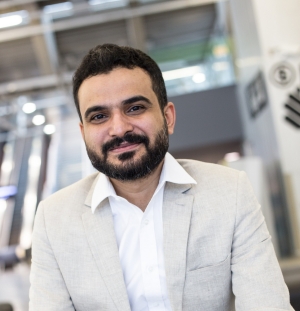
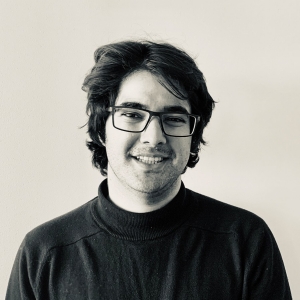
Shahin Masoumi-Verki is a Horizon postdoctoral fellow in the Department of Building, Civil, and Environmental Engineering; and the Concordia Institute for Information Systems Engineering. With a BSc and MSc in Mechanical Engineering, and PhD in Building Engineering, Shahin possesses extensive experience in handling dynamical systems. He has honed his skills in effectively applying data-driven methods to various engineering challenges, particularly Fluid Dynamics. His expertise lies primarily in Reduced-Order Modeling, Sparse Reconstruction, Super-Resolution, Data Assimilation, and Physics-Informed Neural Networks. Shahin adeptly utilizes both experimental and numerical datasets, employing deep learning algorithms and statistical models to analyze and extract valuable insights.
Former Postdoctoral fellows
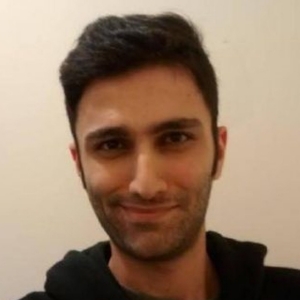
Milad Aghamohamadnia is a Postdoctoral Fellow in the Department of Building, Civil, and Environmental Engineering. His work draws on his background in statistics, data analysis, and machine learning. He earned Ph.D. in Civil Engineering from NYU, and has priorly earned his Master’s and Bachelor’s in Remote Sensing and Geomatics Engineering from University of Tehran and has worked as Data Scientist at Descartes Labs. Currently, he is developing simulation software for modelling urban transportation. His work also covers modelling and implementing heat radiation between buildings and the environment in an urban landscape.
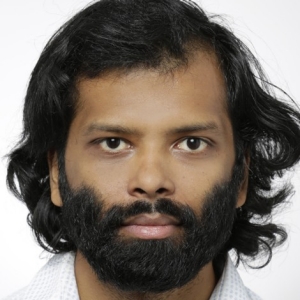
Ramanunni Menon Parakkal is a Postdoctoral Fellow in the Department of Building, Civil and Environmental Engineering. Hailing from Kerala, India, he has previously completed his Ph.D. at EPFL Lausanne on development of predictive control algorithms on polygeneration systems for buildings. His research interests are design and implementation of control systems and frameworks for buildings and the various thermal and electrical systems within buildings, system design, and implementation of IoT infrastructure within the urban ecosystem. His current work is to ensure holistic analysis of user requirements, market models and system limitations to have an efficient and scalable implementation of the smart devices within the next generation of cities.