Artificial intelligence can help get the most out of urban wind energy, say Concordia researchers
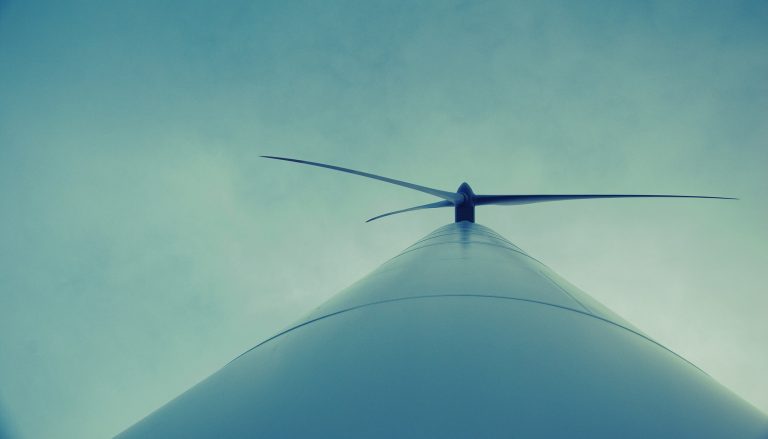
The sustainable cities of the future will rely on an array of energy technology solutions, including some, like urban wind power, that are still in their infancy.
Urban wind power involves the integration of wind turbines into a building’s conception at its earliest stages. And while tomorrow’s designers and architects will labour to come up with the best, most efficient and most attractive way to harness wind, two Concordia researchers have created a tool that could help expedite the task.
That tool is the focus of a new paper published in the journal Building and Environment by Stéphanie Higgins and Theodore Stathopoulos. In it, they present a method that uses artificial intelligence (AI) to assist builders determine where to place their turbines in order to maximize their energy production.
“Using this AI tool can give people an appreciation of how much energy their turbine would generate based on location, wind velocity, building type and other variables,” says Higgins (MASc 20).
“We wanted to see how we could assess different locations of a turbine to see what is more and what is less beneficial.”
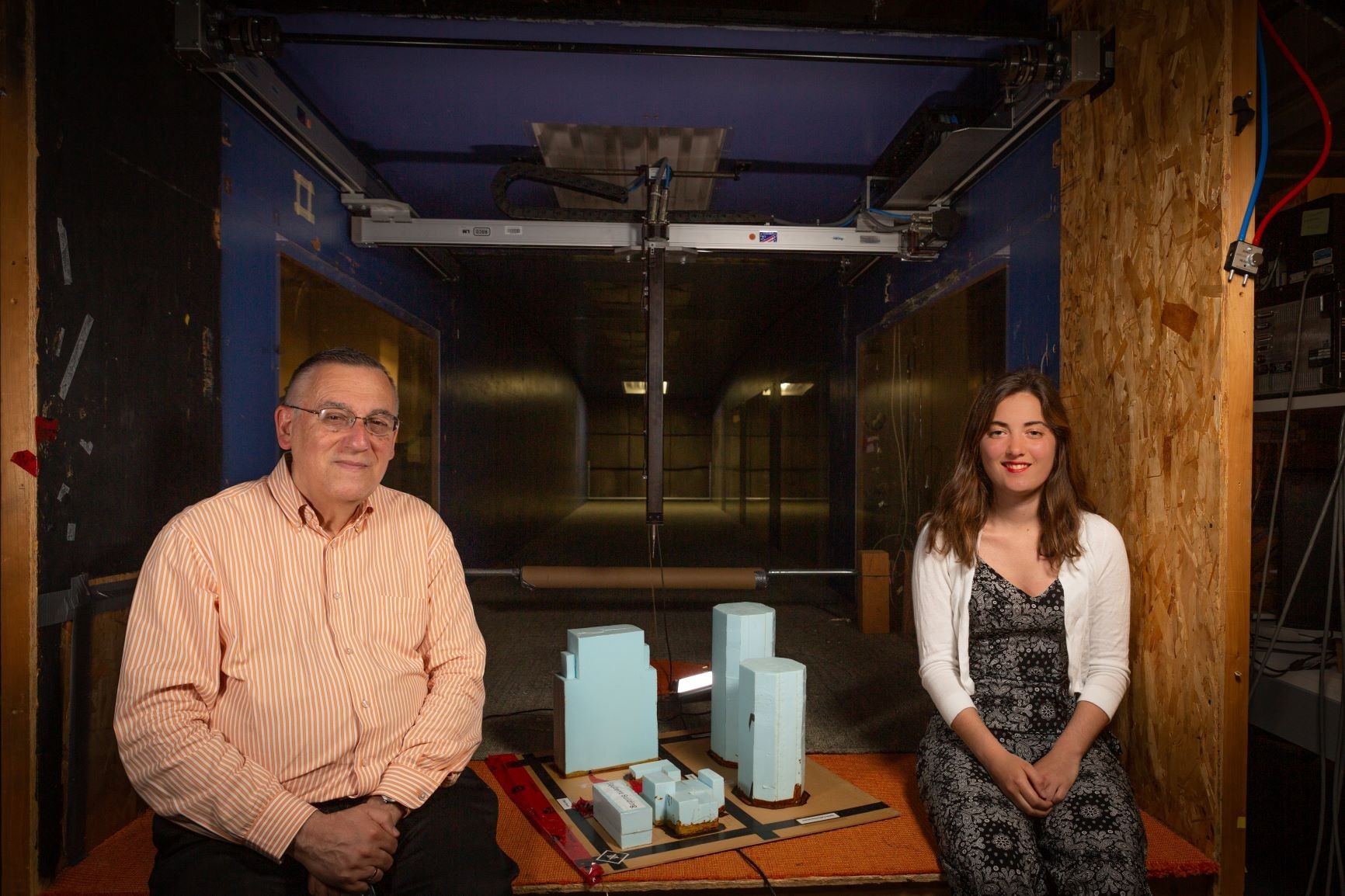
Model velocity
Building on a project she began as an undergraduate, Higgins started the data-gathering process at Concordia’s Building Aerodynamics/Wind Tunnel Lab. It can simulate wind gusts on large buildings with a 1 to 100 or smaller-scale model of a block of downtown Montreal, as well as on individual buildings of different shapes — square, rectangular, U-shaped, T-shaped or L-shaped, and in different configurations. The lab also has a scale model of a section of the Louis-Hippolyte Lafontaine Bridge-Tunnel in east-end Montreal.
“This preliminary work involved a lot of wind tunnel experiments with various building configurations,” explains Stathopoulos, a professor in the Department of Building, Civil and Environmental Engineering at the Gina Cody School of Engineering and Computer Science.
“Stéphanie ran tests for each of them, with wind coming from different directions, as it would in real life, and tried to predict what the amplification of the wind would be at each location. This particular experimentation was interesting because we are trying to see where we can get the highest wind speed. This is the opposite of what we usually do, which is to try to reduce exposure to wind to protect buildings from natural disasters.”
Equipped with information gathered from the existing literature and some 157 cases collected at the lab, Higgins then designed a decisional flow chart and built AI systems trained based on this extensive database. For validation purposes, the researchers ran tests on the systems based on the configurations used previously in the lab. They then compared results with these lab data that had not been used for training of the AI systems. They found that the AI predictive values had up to 99 per cent success rates, meaning that the models often aligned very closely with the values achieved through laborious and time-consuming lab tests.
Gusts of inspiration
The results suggest that AI tools will probably have a major role in future turbine-integrated designs. Turbine placement can have a significant impact on power generation because, the researchers note, wind energy is directly related to the wind velocity cubed. A higher normalized wind velocity will produce a substantial increase in wind energy, so locating a turbine where wind velocity is highest will yield the most energy.
Stathopoulos notes that although the results are preliminary, the overall accuracy of Higgins’s work is encouraging.
“This will be very useful to practitioners,” he says. “We can offer them an asset without doing specific wind tunnel tests for their particular configurations, which would take a lot of time and money. This method, once fine-tuned, can offer them adequate results within a few minutes.”
Read the cited paper: “Application of artificial intelligence to urban wind energy.”