Concordia researchers use deep learning to detect anorexia in social media posts
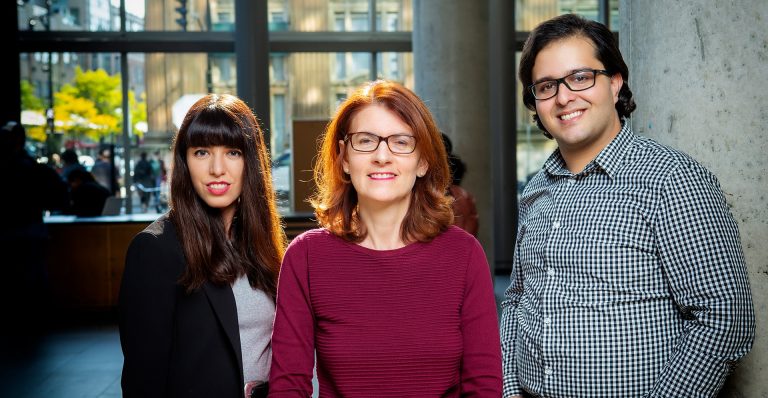
Social media posts are filled with revelatory and often painfully personal information about the individual writing them. However, when these posts are seen in isolation, they may camouflage patterns that reveal the potential presence of serious mental health issues. These can range from suicidal thoughts to post-traumatic stress to eating disorders.
In a paper prepared for the CLEF 2019 conference held in Lugano, Switzerland in September, two Concordia graduate students outline a method of detecting signs of anorexia in individuals by analyzing their social media activity.
The paper was written under the supervision of Leila Kosseim, a professor in the Department of Computer Science and Software Engineering at the Computational Linguistics at Concordia (CLaC) Laboratory.
Elham Mohammadi (MSc 19) and Hessam Amini, who is working toward his PhD, looked at posts by Reddit users labelled anorexic or non-anorexic and a collection of their posts recorded chronologically. Using deep learning algorithms, the researchers looked for patterns of linguistic features in the posts that would identify the individual as being anorexic or at risk of anorexia.
Unlike previous studies, which relied on computational linguists to identify linguistic cues in posts suggesting anorexia, Amini says that “in this system, it is up to the machines and the deep learning algorithms to actually know what the cues are.”
That is, by analyzing enough posts by known anorexic and non-anorexic users, the algorithms will be able to tell the difference between users who suffer from the eating disorder and those who do not. Using widely accepted metrics, their model proved to be highly accurate.
Early detection is key
The authors were far more interested in early detection of mental health issues than detecting harmful behaviour after the fact — something that has so far been under-researched, they say.
“Very often, timing is of the essence,” says Mohammadi. “In suicidal ideation, for instance, we don’t want to detect that after the fact.”
The advantage of using this method is in its reach, explains Kosseim.
“There are so many interactions online, it is just unthinkable to have a human constantly monitoring all of them,” she says. “Detecting posts that suggest anorexia is like trying to find a needle in a haystack. But if you have a machine that monitors and identifies the needle every once in a while, these posts can be forwarded to a mental health professional.”
Balancing needs with privacy
Amini identifies three categories of people who would benefit from this method. The first are those who are personally at risk — the individuals suffering from anorexia themselves.
The second are those who are seeking help for their friends or loved ones. The third are psychologists and other mental health professionals who are researching or treating anorexic patients.
“It is becoming more urgent to identify people suffering from anorexia because studies are suggesting that the number of people in need of treatment is much higher than the number of people receiving it,” says Amini.
The researchers are quick to point out that this model of detection is not designed to replace assessment by a mental health professional. It is meant to complement human expertise.
This naturally brings up questions about privacy and access to the data. The team members observed very strict ethical guidelines. The data they used was provided by an outside agency and was only made accessible to authorized academic researchers.
Kosseim points out that similar systems have been designed to detect cyber-bullying and hate speech on social media. She hopes that mental health professionals will be able to use her research to identify potential victims of anorexia and reach out with help-lines and other forms of online assistance.
The Natural Sciences and Engineering Research Council of Canada (NSERC) provided financial support for this study.
Read the cited paper: “Quick and (maybe not so) Easy Detection of Anorexia in Social Media Posts.”