Seyed Mohammad Mirjalili is a PhD candidate in Electrical and Electronics Engineering at Concordia. He received his Bachelor and Master's degrees in Electrical Engineering from Shahid Beheshti University in Tehran, Iran. Seyed Mohammad is internationally recognized for his contributions to the fields of Evolutionary Algorithms and Photonics. He has published 29 journal articles with over 4000 citations in total, with an H-index of 15 from Google Scholar Metrics.
Ants are probably one of the very first insects that we are exposed to as kids. No one on this planet does not know what ants are, but have you ever asked yourself why? Well, this is because they are everywhere. There are more than 12,000 species of them across the globe.
Ants are special for many reasons:
- they can lift things 20 to 30 times their body weight
- they have painful stings
- they cause billions of dollars in damages to human assets every year
- they have long life spans of up to 30 years
- their jaws can snap at up to 225 kilometres per hour1
- they are mostly deaf and blind
But the most fascinating aspect that has captivated scientists for centuries is their swarming behaviours. An ant colony is one of the most unique biological communities in nature. What makes them special is the fact that each ant is very vulnerable and cannot live individually. There is no centralized control unit to coordinate their activities, but somehow they can do things collaboratively that we cannot even do as humans.
Swarm intelligence
An emerging sub-field of artificial intelligence is swarm intelligence, in which natural intelligence is mathematically modeled and simulated on a computer. The main focus is to understand the collective behaviour and self-organizing mechanisms of particular creatures. Algorithms are developed based on the same principles to solve the problems that we face.
For example, it has been proven that ants tend to find the shortest path from their nest to any food source.2 You have definitely seen them foraging in your leftovers on the ground, a dining room table or even in cupboards. They follow a number of steps to find a food source, mark multiple paths from the nest and choose the best one.
If humans learn those simple steps, we can then solve some of our most challenging problems. For instance, a GPS algorithm finds the shortest path to your destination — it is the same principle. Another example is a logistics company that finds the cheapest path to various destinations to deliver products by multiple vehicles.
Such problems sound simple, but it might take decades for a normal computer program to solve them. Yes, this is not a typo; it might take brute force search techniques, which are often called blind search methods, more than a decade to find the best solutions for such complicated problems. Some of them might even take billions of years.
Therefore, swarm intelligence techniques are essential to solving such problems. This is because they allow a computer to make educated decisions and perform a search more efficiently — just like how natural swarms solve problems.
Natural swarms
Birds, fish, bees, grasshoppers, bacteria and dragonflies also exhibit fascinating natural swarming behaviours. There are many algorithms in the field of swarm intelligence that mimic these creatures for the benefit of humanity.3
Swarm robotics
Swarm robotics is another related area in which simple robots interact to solve problems, just like natural swarms. One issue with current robots is cost. The Curiosity rover on Mars cost around $2.5 billion (USD). A small malfunction in the system could jeopardize the whole project.4
The idea of swarm intelligence is to have a swarm of small and cheap robots instead of one expensive unit. This way, robots can use collective interactions and actions to problem-solve. Losing one robot will not impact the mission. However, it requires very complicated algorithms to achieve certain goals.
We are moving towards micro and nanorobots now, so this field will play a significant role in our lives in the near future.
References
1. S. Yang, Ant jaws break speed record, propel insects into air, biologists find, UC Berkeley News. (2006). https://www.berkeley.edu/news/media/releases/2006/08/21_ant.shtml.
2. S. Goss, S. Aron, J.L. Deneubourg, J.M. Pasteels, Self-organized shortcuts in the Argentine ant, Naturwissenschaften. 76 (1989) 579–581. doi:10.1007/BF00462870.
3. S. Mirjalili, J. Song Dong, Introduction to Nature-Inspired Algorithms, in: S. Mirjalili, J. Song Dong, A. Lewis (Eds.), Nature-Inspired Optim. Theor. Lit. Rev. Appl., Springer International Publishing, Cham, 2020: pp. 1–5. doi:10.1007/978-3-030-12127-3_1.
4. Curiosity (rover), Wikipedia. https://en.wikipedia.org/wiki/Curiosity_(rover).
About the author
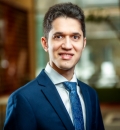